Part 2: Adopting AI Safely & Strategically
Tessa Burg & Patty Parobek
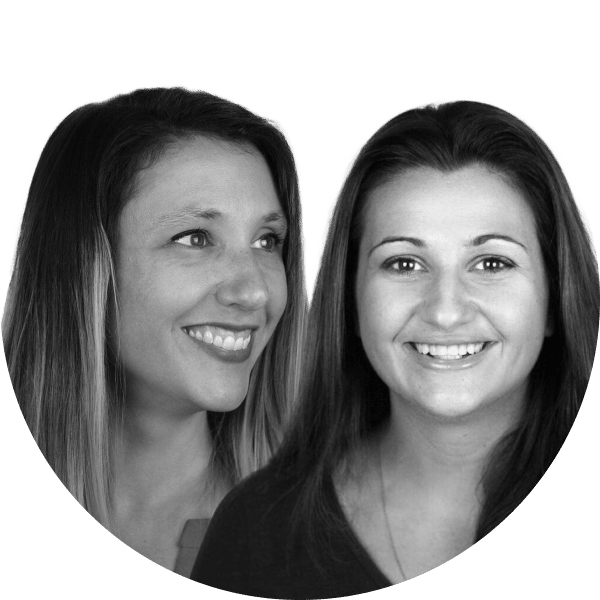
“We want to share this framework because if you can have a shortcut to getting more value out of AI applications responsibly, we want to help you.”
Patty Parobek
Building on part one of the Responsible Use series, Tessa Burg and Patty Parobek explore how organizations can assess AI tools, minimize risks and scale AI-driven innovations effectively.
“With responsible use guidelines, the speed of AI adoption is faster because people know exactly what they can and can’t use AI tools for.”
—Patty Parobek
They share a framework—covering everything from understanding data privacy and compliance to evaluating ROI—designed to empower businesses to adopt AI responsibly while driving measurable value. Listeners will also hear about the “AI Playground,” a fast-track approach to testing AI tools.
Highlights:
- Recap of responsible use policies from Part 1
- The AI application evaluation framework
- Key risks in adopting AI tools: Security, privacy, ethics
- Steps for testing and scaling AI tools
- Insights into the fast-track AI Playground initiative
- Measuring ROI and impact from AI tools
- Common terms and compliance challenges in AI tools
- Governance and centralized IT for safe AI adoption
- Real-world examples of AI innovation
- Predictions for the evolving AI landscape
Watch the Live Recording
Tessa Burg: Hello, and welcome to another episode of “Leader Generation.” I’m your host, Tessa Burg, and this is part two of our responsible use series. Responsible use policy was our AI council’s first deliverable, and it has evolved into a framework that has allowed us to train our staff, use AI responsibly, and build innovations that not only help us deliver work differently for our clients, but also extend into client environments and give their end customers and clients amazing and measurable experiences. I’m joined today by Patty Parobeck. She is our VP of AI Transformation, and she is going to walk you through the framework that we use to operate underneath our responsible use policy, test apps, test our own innovations, measure the value and impact, and show at scale what ROI is for us and our clients. Patty, thank you so much for being here.
Patty Parobek: Yeah, I love being here. I continue to learn more and more as I’m on these different podcasts with you, so thanks for having me.
Tessa Burg: Yeah. I know, it’s fun. I like having another voice in the room, and we’re also gonna be bringing more people to the table, just as a preview. Patty’s gonna walk through our app evaluation framework that helps us execute responsible use, but we’re also gonna touch on a new initiative that came out of this, talking about big ideas, the AI Playground. So stay tuned. Patty’s been on a lot. She’s gonna be on more ’cause AI transformation is a hot topic, but you’ll get a chance to hear from other people within the agency who are participating in the AI Playground and get their direct feedback and insights on what apps they’re using, their thoughts on them, what worked, what didn’t work, and how they compare to other tech in the landscape, because, man, this is a very fast and always evolving landscape.
Patty Parobek: Mm-hm. Absolutely.
Tessa Burg: So with that, Patty. Take us through the app evaluation framework for executing responsible use.
Patty Parobek: Yeah, absolutely. So in the last episode, Tessa covered a lot about responsible use, why it’s important, why governance is so important, why it’s so risky to not have it in place for your organization. And talked a lot about the journey of how Mod Op got to where it is today in the framework that we’ve built to be successful. So know a lot of work went into getting to the place that we are now. And we wanna share that with you because if you can have a shortcut to getting more value out of AI applications responsibly, we want to be the ones helping you with that. So let’s get through what the steps of the framework are. So where we are right now in our learning journey, knowing that this will evolve over time, but at current state, we have an application evaluation framework that’s a 90 day framework. And in this application framework, our first step is assessing the risk level of an application to be able to understand what should the responsible use be, what should the controls be. Based on the vetting framework that we have, should we even allow this application into the organization based on how risky it might be with the clients we have with the work we do? And with that, go into the second step which is getting participants from across the organization, who might really utilize this tool valuably, to help us understand what are the highest and most successful use cases you can find for this tool in a trial period. So as a part of adoption and getting hands into tools of people faster, especially if they’re more hesitant to try, tapping them to participate in a safe and structured evaluation is certainly a way to get skeptics to be like, okay, I can find the use now. ‘Cause, oftentimes what you’ll find is when people are really skeptical, they haven’t used the applications yet. So getting them into a trial is certainly an avenue towards that. In the what is value stage, participants are given responsible use instructions, they sign off on those, and in that phase, they’re telling us, here is the value, here are the use cases I really strongly believe I’ll incorporate eventually or now, and here’s what’s not valuable to me. If we find there’s significant value, we can go into a third phase, which is what is the ROI? ‘Cause if we’re going to scale this across the organization and we found enough use to be able to do that, we certainly need to understand the full impact in ROI over time to justify the expense and to make sure that we have adoption plans, transformation plans, training and skill development plans in place. And, of course, the quality control and governance controls that we need. So how that breaks down, and we’re gonna talk a lot about risk and risk level development because this is where we believe not enough upfront work is probably happening across organizations. In the first episode that you may or may not have heard, only 10% of organizations have responsible use practices in place for their AI policies right now. So this is something to really concentrate on. If you’re in the 90% who hasn’t started creating a responsible use policy, these are things that you need to be aware of. In the risk level assessment, we’re looking at four main categories, security, data privacy, legal and ownership rights, which we’ll talk more about, and then ethical considerations. When we dive into those, and we have a question set that we’ll share with you that we use to assess each one of those, we find where it’s up to snuff and where it’s falling short, okay. So then from there, we can understand based on the risk that it brings in with the places that it’s falling short, what are we going to do for controls, and what are we gonna instruct our team to be able to use this really effectively and responsibly? If you’re wondering what a assessment risk level question set might look like, here are the questions that are mandatory as a part of our assessment framework. For data protection and privacy, is the data protected? Meaning is it encrypted in transit and at rest? Does your data comply with GDPR? You can find that easily in the terms. In fact, you can find questions to 90% of this answered in the terms and conditions of a website and the privacy policy of the website, in the security documentation, and a lot of times, in the Q and A kind of help center hubs of really good applications. And if you are answering all of them, know that that application has done a really great job in providing the answers. But if you’re not finding all of the information there, two things. Number one, assume the application is following on the wrong side of the answer of the question and follow up with a person from the vendor team, like a salesperson, to get the answers that you need. ‘Cause unless you fill out the compliance framework, you’re not gonna know really what your risk level assessment is going to be. That being said, in data protection and privacy, we also have is your input data excluded from training the app’s AI models? By default, a lot of apps just use your data, anything that you put in there to train their models. It’s how they get better and smarter. But if you’re using sensitive data or you intend to use sensitive data, that is something you want to exclude. You don’t wanna lose control over your dataset. So make sure that controls are in place in the application for you to toggle that on and off as you need to. Or that, by default, like Claude, it’s not an option. Do you retain full rights to your input data is another. There’s some times where you input your data, and then you no longer have full ownership over your data. So definitely things to look for in terms. Security and compliance, is access to the app restricted? That’s easy. Does it have a login, right? Even better, does it have a multi-factor authentication? Are there multiple ways that you have to securely log into this application? Because oftentimes, a big part of compliance, security, and data control is just user error. I accidentally left it open on my computer. But if there’s double authentication in place, that happens very, very infrequently. Does the app have security certification? SOC 2 compliance is another one. Does the app follow industry rules necessary for your clients? Like if you are a healthcare institution, does it have HIPAA controls in place if you intend to use PII, PHI in the application. And there are applications, great applications, that are certainly HIPAA compliant in that example. Akkio is one of them. Legal and ownership, do you have full ownership and legal rights to use the outputs, assets or data that comes outta the tool? Are there any restrictions on how you can use that output data? Does the model ensure generated assets don’t infringe on copyrighted content? And ethical considerations, are there formal ethical guidelines governing how the model was designed and how the model is trained? Again, great applications are going to give you exactly what they’re doing. Synthesia is one that does a really great job of telling you exactly how they’re sourcing and exactly how they’re training and designing models with the data that they’re collecting and where. Are data sources used for training legally obtained and licensed? Are artists and creators whose work was used compensated and credited? Artlist.io does a great job with that as well. Is there transparency regarding the app’s environmental impact? This is a, more and more applications are putting out documentation there. But just know, if there’s no documentation that you read on the website, a lot of organizations and vendors that you’ll talk to will give you at least their roadmap on how they’re planning to solve for those things, or at least the regulations that they’re trying to follow or watching. So some examples, just to give you a sense of what you might find in terms when you really start reading through to be responsible in your organization, I’ll go through some popular examples. ChatGPT, it has in its terms that if you don’t wanna use your content to train ChatGPT models, you have to opt out. What that means is, by default, ChatGPT, the free version, anything that you put in is used to train their models. Meaning anything you put in, you will lose control over. They can, and you won’t be able to manage, delete, or prevent the future use of that data. So don’t put sensitive data in the free version of ChatGPT, right? Artlist.io, and I mentioned them before, they’re a creator community. They allow creators to, I don’t, who, what’s a good way of saying this? They’re a community platform where you can build video content based on features and assets and that. So it’s digital asset management for video creation. They have explicitly in their terms that you can’t use any content created from that application, even if it’s mixed with your own content, even if it’s 99% of your own content, in any application or model that learns from user input. That means you can’t use it in your AI machine learning plans. So if you are an organization that’s like, we’re gonna create a bunch of video content, we might use Artlist.io for some of it, and then we’re gonna train this model on video, you can’t do that if you’re using Artlist.io content per their terms. Perplexity is another one. We get a lot of popular use comments on Perplexity. Perplexity is great. I love Perplexity. And know that when you produce content from Perplexity, if it answers a question in a perfect blog format, and you’re like, I wanna publish this blog, you can’t just copy paste the content and publish a blog without citing Perplexity. In fact, you can’t use any parts of the output of Perplexity without citing them or pretending that you created it yourself. ‘Cause explicitly written in their terms is that exact clause. So it’s things to look out for in terms as you’re reading through that make you think based on the work that you do and how you intend on using this tool, what guidance do you need to give your staff? What protections do you need to have in place for your clients, your clients’ data, your clients’ assets that you’re creating? And, you know, what controls do you need to make sure that those are gonna come through to fruition? After a vetting framework is completed for an application, and we understand here are the controls that I need in place based on the things that didn’t necessarily go very well with this vetting framework, or just here is just really good guidance for my team based on how that framework turned out where the questions are that we need a little more maintenance for. We create good instructions for a user group to test out the application. And that’s when we move into the what is the values phase. So now that we have responsible use, guidance, we have controls that we feel like we can monitor, we have a user group come in who’s representative, again, of who all in the organization might use this application long term, and have them start using the application and logging what they found to be effective, what they found to be not so great, if it was valuable, where they might be able to see long-term use. And over that period of time, we’re able to check in with them on are they really understanding responsible use in the way that we intended, and are there things that we need to modify based on their usage? So if they’re using a tool in a way that we didn’t actually intend for them to use, and we hadn’t even thought of that use case and we didn’t protect for it, that’s when we can actually recreate some rules and guidance or even change the risk level to make sure that that compliance and governance is happening. If we find, and this happens a few times, when we find the application is so valuable after the what is value phase that we can even extend it organizationally, we want everyone in the organization to have this tool, or at least most people in the organization to have and use this tool, we’ll move into a what is the ROI and scalability phase, which is we take all of the use cases and amazing documentation of logged use cases with value and do a retrospective around them to find out are these sustainable scalable use cases that would have organizational-wide value, and upon that, what specific metrics are we going to use to estimate out long-term impact? And we’re not talking about time savings as impact. So that does obviously become an impact measurement. We’re talking about growth, increased value, and specific measurements that we can have a real return on our investment for growth. So those measurements we can really only find after knowing how we intend to use the tool and how we intend to use the tool long term and who’s really gonna use it for what. When we start the what is ROI evaluation phase, it’s the same testers, sometimes an expanded set, and they’re doing the same thing. They’re documenting their use cases, but now, they’re doing it with specific measurements in place. So they can say I used it for this use case again, but now it gave me X in increased value versus Y of what it used to be and this much in time savings ’cause of course we still have to track efficiency. In this phase two, we’ll start creating training and education for skill development on use cases if we find a really great tool and know that to be able to use this use case most effectively, we have to increase the aptitude of, I don’t know, data literacy. We have to increase the aptitude of creative thinking. We have to create just the aptitude using the tool itself and navigating the features. That will go into our AI academy as well as how to use the tool responsibly. So if there’s gotchas in that vetting framework, those are incorporated with how to navigate those. IT support and license management also happens. So something I didn’t mention that I should have very early on is we don’t have people go kind of like download tools themselves. It’s a part of our process that we use our centralized IT team to assign user access for multiple reasons. One, it’s very easy to manage subscriptions. That way if someone stops using the tool, it’s really easy to sunset if they’re not finding value and it controls cost. But another reason is because it’s a control in place for risk. If your risk goes from a level one risk to a level three risk, meaning like goes from a very okay, not very risky to very risky application ’cause terms do change, so this is not a one and done kind of framework, then you can easily pause access until you figure out next steps. So license management being centralized is really important. And this is in that phase where that can start being planned for in a scalable way as well. And this is a great framework, but my goodness, there’s a lot in it, right? And it’s 90 days, and maybe that doesn’t sound like a lot to you if you’re a very large enterprise who’s used to 90 day sprints or 90 day projects. But if you’re a small organization and wanna move more quickly, how are you going to get tools in the hands of your users more quickly? And that’s where you can use an open concept kind of experimentation fast track into the process. We call ours the AI Playground. And in this fast track process, we follow the same controls. It’s the same framework, But the access to tools happens within 48 hours. So a user will identify, I wanna use this new X, Y, Z tool for AI that came out. I have this use case for it. This client gave me explicit permission already, so I know that I can use their data. So what, how can I get access immediately? I want the paid version. So they’ll submit that to us. We’ll do quickly the risk level assessment. And quickly doesn’t mean we’ll skip steps, it just means we’ve gotten so efficient at doing 400 plus of these, we know exactly where to look for things and how to pull them out. And then we’ll give them responsible use guidance with all of the considerations that they’ll sign off on. And then they’ll start doing their log of use cases and value on their own. And then if we see multiple people coming and signing up for the same tool, then we can formally kind of move them into the formal process, which is longer, but it’s parallel path to then getting the tools and using them right away. So, again, it sounds cumbersome, but when you’re building brakes, it does lead to faster acceleration, right? So that process, and it was a journey getting there, it works. And our speed of adoption is so much faster because people know exactly what they can and cannot use a tool for. So there’s no pause and hesitation, and they’re diving right in. The speed to impact eight to five use cases is just our starting point. We expect that to increase as people get really familiar with AI tools in general as they’re testing out new things, especially if they’re like tangential platforms, if they do kind of the same thing in similar ways. And then speed to innovation, we’ll continue to see gaps. We’re testing 25 to 30 apps right now. Not a lot of them will work. And maybe that is a hole that we can fill and build upon in our own innovations knowing that our clients will need something better. And that is our framework.
Tessa Burg: Yeah. Yeah, that was a lot! So I just wanna highlight some really big takeaways. One, 400 apps have gone through our responsible use framework, our app evaluation process. And to reiterate what I said in episode one, there are 67,200 different AI startups right now. I mean, I remember when we used to track the marketing tech landscape, and there’s like 14,800. All 14,000 plus of those marketing applications will have AI in it. All 67,200 companies that identify as AI obviously have AI in it. So if you haven’t already, read the terms and conditions, look for those gotchas, make sure you know how the tech that you’re using today is accessing your data, processing, and what is the output? Where is it going? Is it training their model? What is the quality of the output? How do you identify hallucinations, validate accuracy? These are all incredibly important questions. And I love the emphasis on the results. I know these are a lot of steps, and if you don’t know where to get started and you have not done an audit yet, yes, we can certainly help you. We’ve gotten ridiculously efficient at this. We’re now scaling it at our own company using tools that we’ve built. We do offer extensions of those tools and our platform. We have our own proprietary transformation platform because what we found is general training doesn’t cut it. Your business is unique and different. Whether you’re B2C or B2B, you have your own vision, values, and the value prop that you have for your products and services needs to be protected as well as it needs to be transferred into how you build your training and your career paths for your staff, so that as you elevate them to strategic thinkers and problem solvers, they are doing so in a mutually beneficial way that helps scale your overall business for your end customers. And they’re gonna ask questions. They’re gonna want to know how are you training? How are you protecting me? Was this created by AI? So a lot here to unpack. You can find these visuals on our website, modop.com. We have our responsible use policy published there. It’s super duper simple, super short. We do not use generative AI for any client outputs, just to address the number one question that we get. But are we using generative AI? Absolutely. It is giving us predictive insights. It’s helping us better understand our clients’ target audiences. It’s helping us make sure we get the right message to the right person at the right time and create unparalleled efficiencies that we simply haven’t been able to do in the past. And we didn’t like spending all that tedious time and work doing manual, no value add activities. So it’s had a massive impact on our business. We wanna help have a massive impact on your business in a safe way. Go to modop.com. You can listen to more “Leader Generation” episodes. If you have a question for us, specifically on responsible use or really anything related to AI or any of the podcasts for that matter, you can email us at [email protected]. That’s P-O-D-C-A-S-T-S, I think it’s podcasts plural at mod, or maybe, no. No it’s not. It’s podcast singular at modop.com. So [email protected]. Ask us a question. And until next time, thanks, Patty, for once again joining us. We appreciate it.
Patty Parobek: Always happy to be here and looking forward to being here more often.
Tessa Burg: Yay! All right, we’ll talk to you all later. Have a great week.
Tessa Burg & Patty Parobek
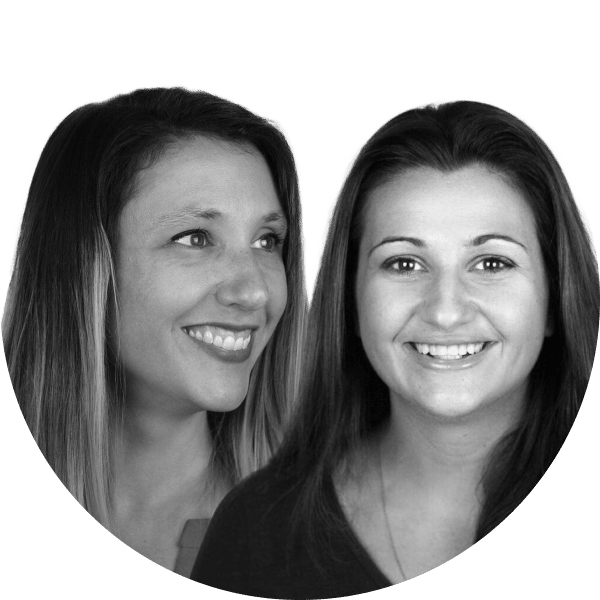
Tessa is the Chief Technology Officer at Mod Op and Host of the Leader Generation podcast. She has led both technology and marketing teams for 15+ years. Tessa initiated and now leads Mod Op’s AI/ML Pilot Team, AI Council and Innovation Pipeline. She started her career in IT and development before following her love for data and strategy into digital marketing. Tessa has held roles on both the consulting and client sides of the business for domestic and international brands, including American Greetings, Amazon, Nestlé, Anlene, Moen and many more. Tessa can be reached on LinkedIn or at [email protected].
As Vice President of AI Transformation, Patty leads Mod Op’s AI practice group, spearheading initiatives to maximize the value and scalability of AI-enabled solutions. Patty collaborates with the executive team to revolutionize creative, advertising and marketing projects for clients, while ensuring responsible AI practices. She also oversees AI training programs, identifies high-value AI use cases and measures implementation impact, providing essential feedback to Mod Op’s AI Council for continuous improvement. Patty can be reached on LinkedIn or at [email protected].